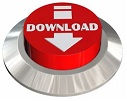

Much less work has been done on the effects of heteroscedasticity on these tests all I can recommend is that you inspect the data for heteroscedasticity and hope that you don't find it, or that a transformation will fix it. Homoscedasticity is also an assumption of other anovas, such as nested and two-way anovas, and regression and correlation. This means that non-parametric tests are not a good solution to the problem of heteroscedasticity.Īll of the discussion above has been about one-way anovas. Non-parametric tests, such as the Kruskal–Wallis test instead of a one-way anova, do not assume normality, but they do assume that the shapes of the distributions in different groups are the same. The most commonly used alternative to one-way anova is Welch's anova, sometimes called Welch's t–test when there are two groups. If the standard deviations of your groups are very heterogeneous no matter what transformation you apply, there are a large number of alternative tests to choose from (Lix et al. It's best if you can choose a transformation based on a pilot study, before you do your main experiment you don't want cynical people to think that you chose a transformation because it gave you a significant result. A common pattern is that groups with larger means also have larger standard deviations, and a log or square-root transformation will often fix this problem. If you see a big difference in standard deviations between groups, the first things you should try are data transformations. However, despite all of the simulation studies that have been done, there does not seem to be a consensus about when heteroscedasticity is a big enough problem that you should not use a test that assumes homoscedasticity. You should always compare the standard deviations of different groups of measurements, to see if they are very different from each other. The problem of heteroscedasticity is much worse when the sample sizes are unequal (an unbalanced design) and the smaller samples are from populations with larger standard deviations but when the smaller samples are from populations with smaller standard deviations, the false positive rate can actually be much less than 0.05, meaning the power of the test is reduced (Glass et al. 1972), but later results found that large amounts of heteroscedasticity can inflate the false positive rate, even when the sample sizes are equal (Harwell et al. Early results suggested that heteroscedasticity was not a problem at all with a balanced design (Glass et al. Heteroscedasticity is much less of a problem when you have a balanced design (equal sample sizes in each group). There have been a number of simulation studies that have tried to determine when heteroscedasticity is a big enough problem that other tests should be used. In other words, even though the population means were really all the same, my chance of getting a false positive result was 18%, not the desired 5%. However, when I made the standard deviations different (1.0 for population A, 2.0 for population B, and 3.0 for population C), I got a P value less than 0.05 in about 18% of the simulations. When the three populations were homoscedastic (had the same standard deviation), the one-way anova on the simulated data sets were significant ( P<0.05) about 5% of the time, as they should be. I simulated taking samples of 10 observations from population A, 7 from population B, and 3 from population C, and repeated this process thousands of times. To illustrate this problem, I did simulations of samples from three populations, all with the same population mean. If the standard deviations are different from each other (exhibit heteroscedasticity), the probability of obtaining a false positive result even though the null hypothesis is true may be greater than the desired alpha level. One of the assumptions of an anova and other parametric tests is that the within-group standard deviations of the groups are all the same (exhibit homoscedasticity).
#Tests for homoscedasticity in xlstat how to
Here I explain how to check this and what to do if the data are heteroscedastic (have different standard deviations in different groups). Parametric tests assume that data are homoscedastic (have the same standard deviation in different groups).
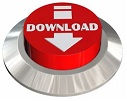